Model Risk: How to Cope with the Volatility of Loss Estimates
8/3/2021
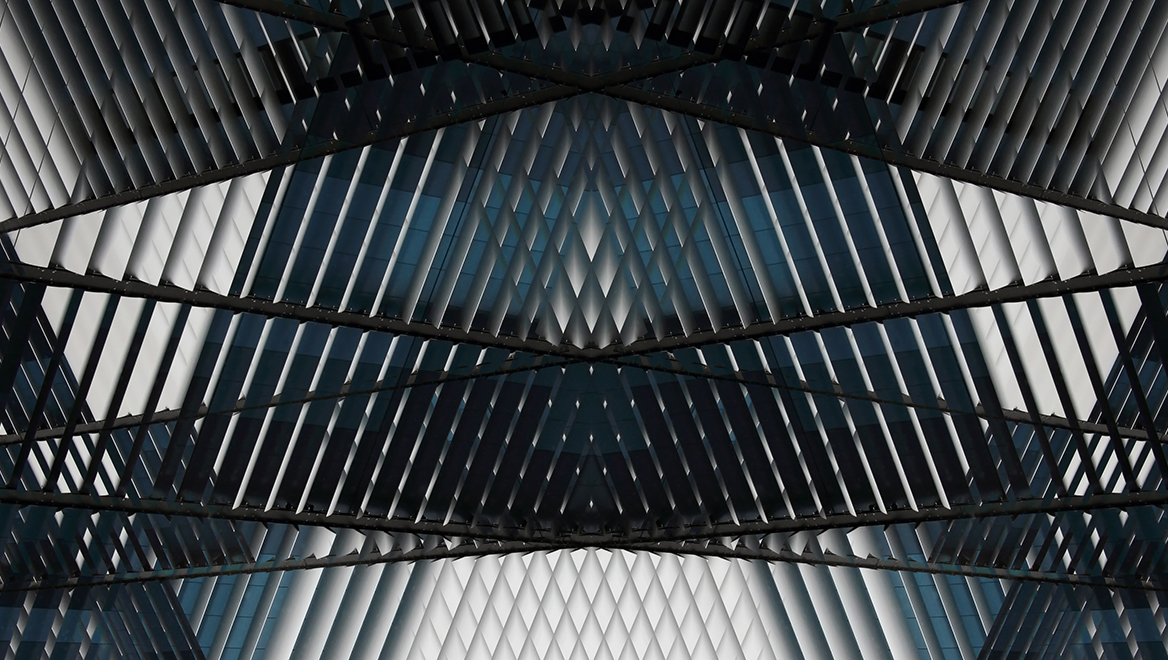
Identifying potential issues with loan portfolios has been an everlasting challenge for portfolio managers and risk professionals. Most recently, the COVID-19 pandemic proved to be a real test when loan loss estimates escalated quickly at the onset of the pandemic.
Businesses were impacted by state-wide shutdowns and other government responses to the public health crisis. Stimulus policies eliminated some of credit losses that lenders were anticipating. Yet, lenders may face hidden risks due to the managements’ loss estimates and modifications.
In the following interview with Stephen Krasowski, Communications Manager at RMA, two subject matter experts on financial risk management from KPMG, Benjamin Hoffman and Joseph Bielecki, discuss why there is volatility when estimating loan loss provisions and what lenders may see in terms of COVID-related modeling modifications and challenges. The information is derived from the interview.
RMA: Credit characteristics of the portfolio may have an impact on the estimate of credit losses if loss estimation processes are not adjusted to address the impact. What are some of the challenges from these modifications?
Bielecki: I think the biggest challenge of these modifications is that it creates the potential for hidden risks. In other words, if a modification has existed for an extended period on a given loan, should that loan really be treated as current just because a modification was granted, or should it be looked at as if it is 180 days passed or potentially more from a loss estimation perspective? How do we think about the underlying financial statements of the borrower, especially if it is a commercial type of asset? Do we think that they are going to have the ability to recover from this crisis or do we think that they are going to continue to struggle?
It could affect characteristics such as those made in segmentation decisions where previously things with perhaps, light collateral, may have been grouped but now you need to also consider what industry that business is operating in.
So, [the challenges are] thinking about all those things where you made assumptions and you made decisions in creating a CECL process in saying this is how we're going to estimate CECL and revisiting literally all of those decisions.
RMA: The volatility in economic forecasts could have a significant impact on CECL estimates. Could you talk a little bit about this and what banks can do to prepare for uncertainty in their forecasts?
Hoffman: I think the biggest opportunity to prepare for this volatility is the robust scenario and stress testing analysis capabilities and looking at a wide range of scenarios. Some people might look at [certain] scenarios through their models, but there might also be other variations on those scenarios to really understand what the potential range of outcomes is and how that volatility might occur quarter over quarter, depending on the sequence of events that occur.
Within those scenarios, [it is about] the ability to drill down into the key drivers of the estimates to understand what is driving those differences across the scenarios relative to what is going on in the underlying portfolio. Some of those sources of imprecision that might necessitate a qualitative adjustment, either positive or negative, might be some of the deferrals and what is going on with them that we have been talking about.
How much of the NOI [Net Operating Income] and other deterioration is already reflected in risk ratings - to the extent an organization that is using risk ratings - or those metrics themselves really drive management's understanding of the impact that those scenarios might have to rewind a couple of quarters. Many of those values may still be 12 [December] 31 numbers and reflect a stable, slightly growing economy. Over time more of those are being replaced with post-COVID numbers.
Well, you are double counting the impact of the adverse scenario because if you're using pro forma numbers, you're already capturing that as well. So, how does management think about those types of risks as they're going about both capturing on an individual ratings and financial metric level, but also using the forecast provided by the vendor or internal team. So, qualitative adjustments may be necessary to better align the loss forecast with the true level of risk in the portfolio.
The other way we have seen banks approach the volatility gets down to using multiple scenarios. Many institutions use a single scenario approach from a less complex perspective at the [CECL] transition date.
We have seen many switching to either directly using multiple scenarios or implicitly applying qualitative factors based on multiple scenarios and then tweaking the weights of the different scenarios as we move to the year to reflect information that may be subsequent to the development of the forecast but prior to the end of the financial reporting period. So, we see a lot more activity in how banks think about the scenarios and what are the appropriate ones to use to help them create the best estimate facing that uncertainty.
RMA: So, moving on to modeling, how are modeling limitations affecting seasonal estimates and what could banks do about that?
Bielecki: Well, if you think about it, models are always based on data. That data comes from our history. These are things that have been historically observed. We have not in the history of this being used by these banks since at least the early 1900s seen something like what we are seeing with COVID and that creates a model limitation.
Further, the magnitude and speed of change hasn't really been in existence in those last 10-, 15- and 30-year periods. Generally, you see a recession happen with a steep incline.
Let's say, for unemployment rate, where things devolve quickly and then you see an improvement that takes a fair amount of time to achieve, you know, generally two to three years. Those changes, even in the historical data points of 2020, are still affecting our estimates at 2020 year-end and 2021 due to lead/lag relationships that might be used.
So, we need to think about the volatility that those rapid changes are creating in our model estimate. Are they causing our probability of default to rapidly increase to levels that are above expectations and then also rapidly decrease as it looks like unemployment's massively improving, but in fact, it's just better capturing a very temporary effect?
It also reduced our ability to utilize some of those variables that were always dependable in historical modeling, like unemployment, like changes in GDP. Those have always had a strong relationship with [loan] loss. But due to the stimulus, we are not seeing that. We need to look to alternative models, perhaps with different variables that have remained steadier and better capture our thoughts on risk of loss in this environment.
So, those rapid and significant changes, as well as the stimulus package, is just creating a set of circumstances that is confusing models and potentially requiring qualitative adjustments and/or management overrides, which can be very significant and they can also be both positive or negative depending on circumstance.
RMA: COVID-related impacts are creating a need to revisit certain accounting policy, elections and assumptions. What are some of these elections and assumptions?
Hoffman: There's a couple of different things that come to mind here. Certainly, [we] talked a lot about the relevance of historical data in the post-COVID world, thinking about they are going to have a lot of attention on things like probability default and loss given default. But there are a lot of other assumptions, prepayment rates and extension considerations that may have very different expectations of behavior in a post-COVID world than they did before.
Maybe for some portfolios and some segments, it's very similar, but those need to be reevaluated and rethought through to make sure they remain valid post-COVID. The second one we talked a little bit about this earlier, the shift from single to multiple scenarios and how institutions think about managing that risk and why those assumptions make sense.
There really needs to be a robust rationale for what is driving those decisions and that can be challenging for organizations. The third thing that comes to mind is a lot of institutions have elected to reverse accrued interest in a timely manner, which makes sense in a precommitment world when I think of post-COVID and all the deferrals. Is there a risk, particularly across financial reporting periods where you have deferred a lot of interest and the borrower is still not paying?
RMA: Controls and governance are some of the most important aspects of financial statement preparation. How important are controls in identifying and tracking COVID-related modifications in seasonal estimates?
Bielecki: Well, we've already talked quite extensively about the the complexities and the complications that modifications and the environment as a whole have brought to the estimate. What's really necessary is management judgment. Management judgment and overrides. It might be used in qualitative factors and it could be used in decision making, including any of the policy decisions or key assumptions like prepayment that Ben just discussed. Any time you're executing changes, governance is necessary.
But when those changes rely on judgment, it's even more so necessary. So one of the really important things for all financial statement preparers to understand is they address the standard. In the current environment, those estimates might require a higher level of judgment, a more impactful judgment. The magnitude of the impact could be potentially much more than materiality. And so those governance controls and the activity level controls that make sure that the individual calculations that support these judgments are accurate because they become much more impactful and much more important purely because of the extent of judgment and the impact of judgment in addressing these unique coronavirus related circumstances.
RMA: To wrap up, what can banks do now to prepare for the transition to CECL in such a volatile environment?
Hoffman: For the banks that have not transitioned already, I think it is a lot of the same advice you talked about earlier. How to manage that volatility, the ability to think about different scenarios and model out those different outcomes to understand the impact on the portfolio, thinking about different horizons and how that might drive outcomes, particularly things that might not really have thought about before in terms of maturities of assets and the impact that CECL has on long-dated assets relative to short-dated assets compared to the incurred method.
SUMMARY:
- Understand the different levers that impact your bank’s current loan loss estimates.
- Know the underlying assumptions both in an isolation and in contexts.
- Test those assumptions and models to ensure their performance.
LEARN MORE
Meet our team at MVC by RMA to discover customized model validation solutions for you.
Stay tuned for upcoming RMA podcast episodes.