Model Risk: Follow the Way Fraud Models Scale
6/1/2022
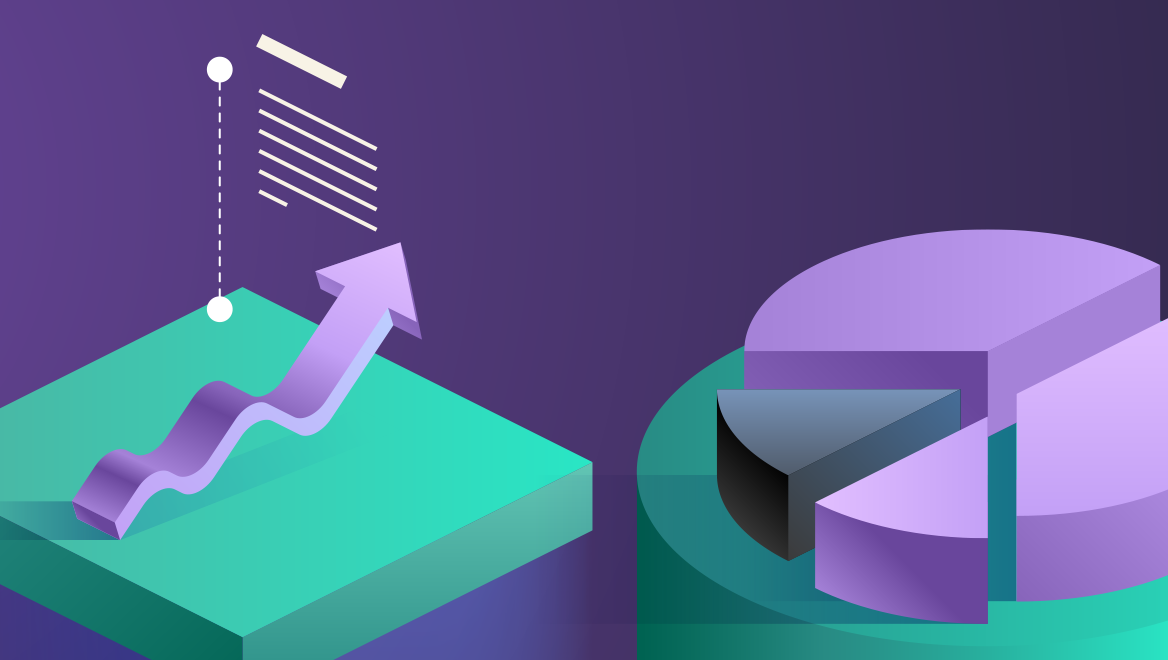
Due to the effectiveness of pattern recognition, many banks leverage machine learning models for their fraud prevention and detection systems. However, models are not perfect, and they often fail. As ML models generate more false positives, banks must devote additional time to conduct manual investigations to confirm outputs, thus increasing costs and risk.
Regulators are also paying more attention to new modeling techniques and their risk implications. The Office of the Comptroller of the Currency published the Model Risk Management Comptroller’s Handbook in August 2021. The handbook provides the OCC’s latest observations on model risk management practices in the financial services industry, including AI/ML models, non-model governance, and validation.
According to the handbook, regardless of how AI/ML is classified by regulated entities, the associated risk management should be commensurate with the level of risk of the function that the AI and ML support.
An RMA survey showed that the regulator’s observation inspired survey respondents to rethink their definitions of models, particularly for AI and ML-related models and tools. The survey also revealed that of the respondents have considered rethinking their model risk governance framework for non-model tools.
Source: RMA Survey of Model Risk Management, Vendor Model Validation, and Third-Party Model Risk Management (2022)
Source: RMA Survey of Model Risk Management, Vendor Model Validation, and Third-Party Model Risk Management (2022)
The fraud prevention model failure is critical for lenders, due to the scalability of an ML model once it is deployed. The more data available for the algorithms, the more they learn with improved precision.
Technological advancement accelerates the sophistication of these models every day. What are the best practices in AI/ML model validation for lenders? Speak with us to learn more about the survey results and what your peers say about utilizing AI/ML models for fraud prevention and detection.