With Increased Bank Usage of AI/ML Models, Risks Arise
7/27/2023
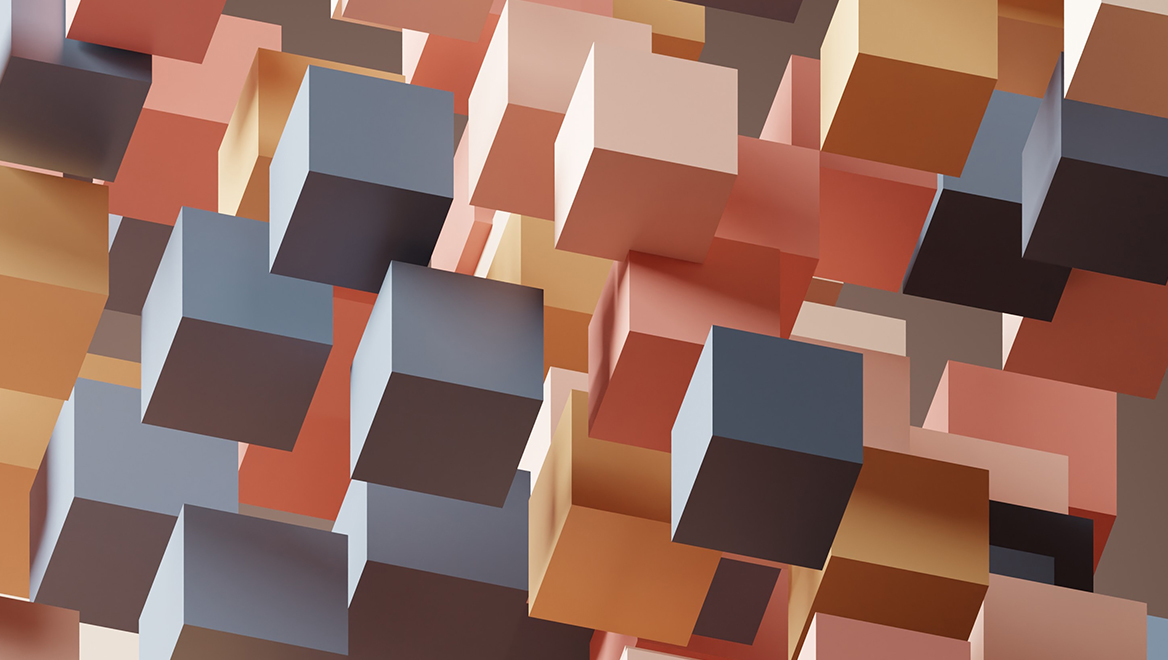
‘Sometimes the statistical relationships that surface are intuitive, but often they are not. As a result, to many users, AI/ML models seem like ‘black boxes.’’
Seventy-three percent of banks report using artificial intelligence or machine learning models and tools, according to a new RMA survey report. The widespread adoption of AI/ML, however, has raised questions about how such models reach their conclusions and whether those conclusions are fair and sound.
“The Risk Management Association Survey of Model Risk Management,” released this month, showed an overwhelming majority of those institutions use AI/ML—84%—for fraud detection. Marketing was a distant second (41%), followed by underwriting (32%), and customer interaction (30%).
“AI/ML models are particularly useful for finding patterns in masses of data,” explained RMA risk consultant Thomas Gregory, an architect of the survey. “That’s why fraud detection is the most common use case. It’s similar for marketing and underwriting, particularly in the retail markets where there are many customers and the need for efficient screening and scoring processes.”
Unlocking the ‘black box.’ Banks do, however, have understandable concerns about leaning too heavily on AI models. Top issues listed by survey respondents included explainability, theoretical and conceptual soundness, fairness, and interpretability.
“AI/ML models don’t start from the top-down with a strong set of theories of what drives outcomes,” Gregory said. “Rather, they simply seek out the patterns that exist in the data. Sometimes the statistical relationships that surface are intuitive, but often they are not. As a result, to many users, AI/ML models seem like ‘black boxes.’”
Bad role models. Some worry that AI-powered models, if not trained on the proper data, can perpetuate biases in lending instead of eradicating them.
“Early on, it became evident that models that are calibrated or ‘trained’ on past decisions can actually replicate the bias of the prior human assessors,” Gregory said. “Ethically, these are biases that we need to overcome. Fair banking regulations also require it. So model validation processes have been expanded to include testing to detect biases that could be based on geography, race, religion, national origin, sex, marital status, or age, among other things.”
Download the report executive summary here.